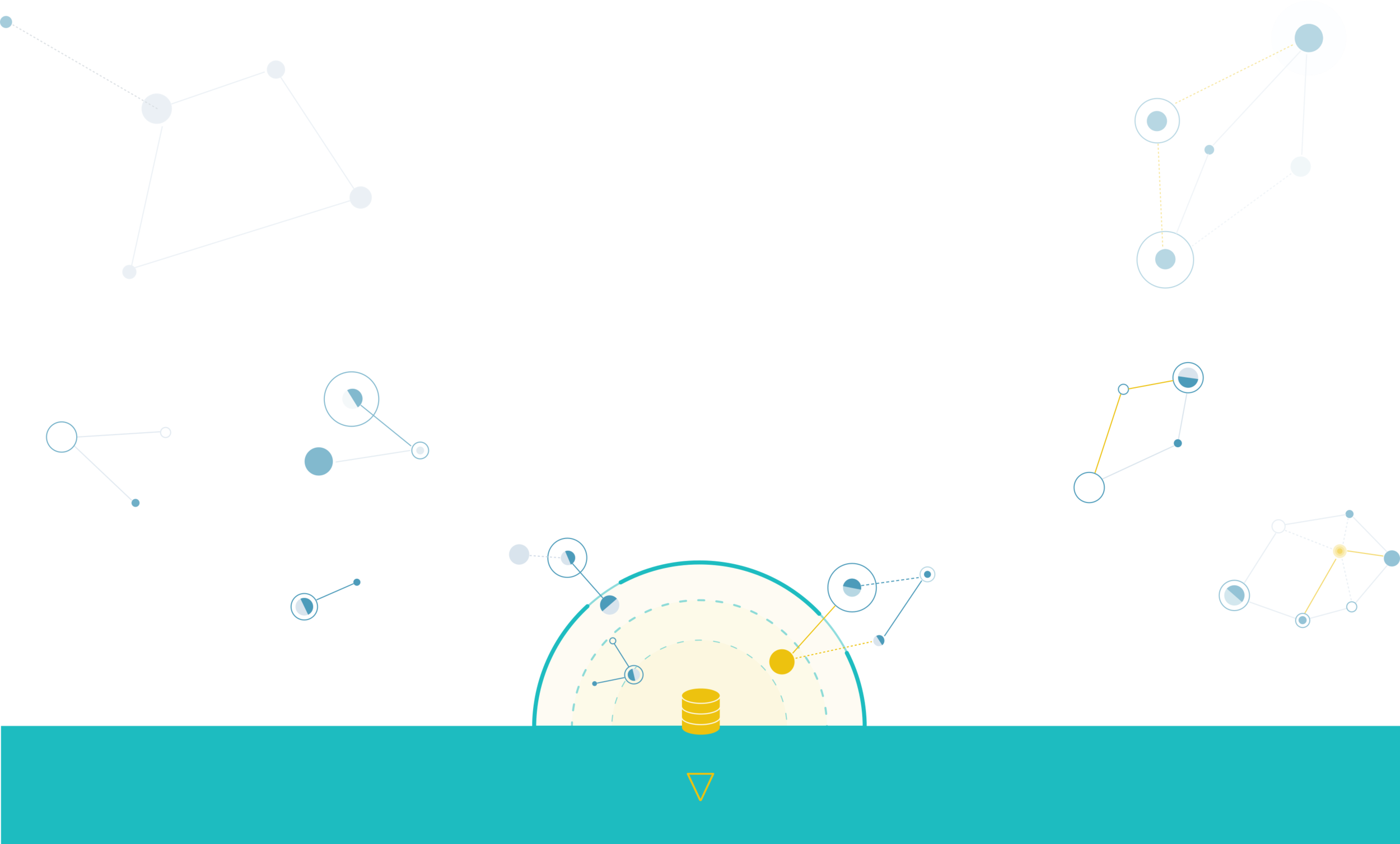
Towards trustworthy data science
AI technologies require massive amounts of data to achieve high predictive performances. The circulation, processing and compilation of datasets by multiple actors raises concerns about privacy issues and risks of sensitive information leaks. Further, many in the tech industry, research sector, and public organizations are growing more preoccupied with AI standards, such as how algorithms are trained and tested, and how to measure the significance and robustness of AI performance. As of today, with clear risks and work-in-progress regulations, it is difficult to trust AI.
Simultaneously, ML and data science keep expanding into research, business processes, marketing and advertising, products and services in countless industries. Whether presented as new techniques, specialised tools, or general capabilities, this diffusion of ML fuels a multiplicity of projects aimed at gaining new insights and opens up many possible fields where AI could stimulate innovations. The potential of AI is immense.
The two trends, the growing concerns about privacy, transparency and quality of AI, and its expansion into many sectors and organizations, won’t disappear in the coming years. We believe that we need to address both, and in reconciling and combining them we can foster a sound development of trustworthy AI. New technical and organizational solutions are required for this endeavor, to build up trust, to enable large scale collaborations of citizens, companies and institutions; ultimately, to create the conditions for responsible, privacy-preserving, and quality data science. In short, trustworthy AI by-design is needed, and Substra Foundation is entirely committed to contributing to it.
As of today, it is difficult to trust AI
The potential of AI is immense
Trustworthy AI BY-DESIGN is needed
We are an independent non-profit organization dedicated to fostering the development of trustworthy data science ecosystems (Learn more about us).
Secure, traceable, distributed ML orchestration
Different privacy-enhancing technologies are being developed by the privacy community and tested by a growing number of interested parties. They contribute to advancing the options for reinforcing the privacy of datasets and models in data science projects, and are becoming increasingly instrumental.
Substra framework is a low-layer tool, offering secure, traceable, distributed orchestration of machine learning tasks among partners. It aims at being compatible with privacy-enhancing technologies to complement their use to provide efficient and transparent privacy-preserving workflows for data science. Its ambition is to make new scientific and economic data science collaborations possible.
Data locality
Data remain in their owner's data stores and are never transferred. AI models travel from one dataset to another.
Decentralized trust
All operations are orchestrated by a distributed ledger technology. There is no need for a single trusted actor or third party: security arises from the network.
Traceability
An immutable audit trail registers all the operations realized on the platform, simplifying certification of models.
Modularity
Substra framework is highly flexible: various permission regimes and workflow structures can be enforced corresponding to every specific use case.
Ongoing projects and consortiums
AI on clinical data
The HealthChain consortium gathers French hospitals, research centers and start-up organisations together with Substra Foundation to develop AI models on clinical data. Substra framework enables the training and validation of AI models and, in doing so, secures the remote analysis of sensitive data. This project will provide the first proof of concept of the Substra framework and will prove its compliance with GDPR.
(9 partners, 10M€ funding)
More details
Drug discovery
The MELLODDY project aims to develop a platform for creating more accurate models to predict which chemical compounds may be most promising in the later stages of drug discovery and development. It demonstrates a new model of collaboration between traditional competitors in drug discovery and involves an unprecedented volume of competitive data. The platform aims to address the need for security and privacy preservation while allowing for enough information exchange to boost predictive performance.
(17 partners, 18M€ funding)
More details